Inside Microsoft Copilot: A Look At The Technology Stack
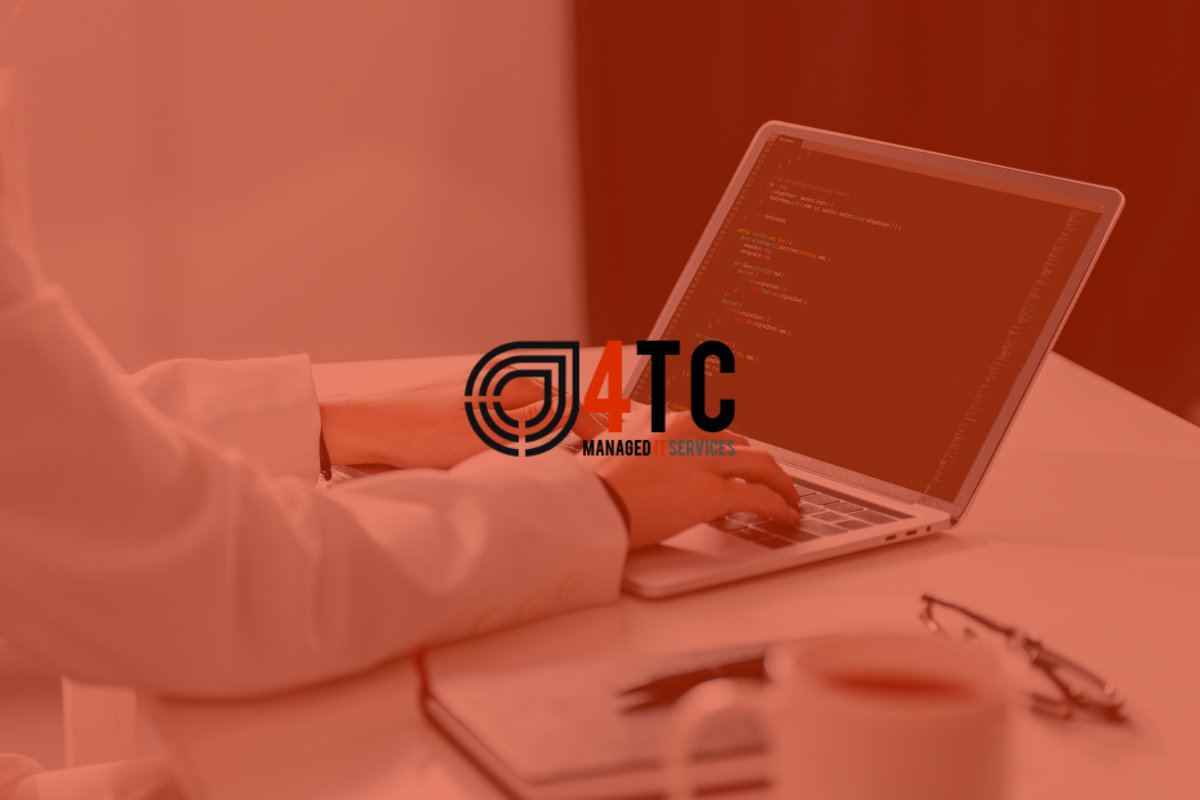
As expected, generative AI took centre stage at Microsoft Build, the annual developer conference hosted in Seattle. Within a few minutes into his keynote, Satya Nadella, CEO of Microsoft, unveiled the new framework and platform for developers to build and embed an AI assistant in their applications.
Branded as Copilot, Microsoft is extending the same framework it is leveraging to add AI assistants to a dozen applications, including GitHub, Edge, Microsoft 365, Power Apps, Dynamics 365, and even Windows 11.
Microsoft is known to add layers of API, SDK, and tools to enable developers and independent software vendors to extend the capabilities of its core products. The ISV ecosystem that exists around Office is a classic example of this approach.
Having been an ex-employee of Microsoft, I have observed the company’s unwavering ability to seize every opportunity to transform internal innovations into robust developer platforms. Interestingly, the culture of “platformisation” of emerging technology at Microsoft is still prevalent even after three decades of launching highly successful platforms such as Windows, MFC, and COM.
While introducing the Copilot stack, Kevin Scott, Microsoft’s CTO, quoted Bill Gates – “A platform is when the economic value of everybody that uses it exceeds the value of the company that creates it. Then it’s a platform.”
Bill Gates’ statement is exceptionally relevant and profoundly transformative for the technology industry. There are many examples of platforms that grew exponentially beyond the expectations of the creators. Windows in the 90s and iPhone in the 2000s are classic examples of such platforms.
The latest platform to emerge out of Redmond is the Copilot stack, which allows developers to infuse intelligent chatbots with minimal effort into any application they build.
The rise of tools like AI chatbots like ChatGPT and Bard is changing the way end-users interact with the software. Rather than clicking through multiple screens or executing numerous commands, they prefer interacting with an intelligent agent that is capable of efficiently completing the tasks at hand.
Microsoft was quick in realizing the importance of embedding an AI chatbot into every application. After arriving at a common framework for building Copilots for many products, it is now extending to its developer and ISV community.
In many ways, the Copilot stack is like a modern operating system. It runs on top of powerful hardware based on the combination of CPUs and GPUs. The foundation models form the kernel of the stack, while the orchestration layer is like the process and memory management. The user experience layer is similar to the shell of an operating system exposing the capabilities through an interface.
Let’s take a closer look at how Microsoft structured the Copilot stack without getting too technical:
The Infrastructure – The AI supercomputer running in Azure, the public cloud, is the foundation of the platform. This purpose-built infrastructure, which is powered by tens of thousands of state-of-the-art GPUs from NVIDIA, provides the horsepower needed to run complex deep learning models that can respond to prompts in seconds. The same infrastructure powers the most successful app of our time, ChatGPT.
Foundation Models – The foundation models are the kernel of the Copliot stack. They are trained on a large corpus of data and can perform diverse tasks. Examples of foundation models include GPT-4, DALL-E, and Whisper from OpenAI. Some of the open source LLMs like BERT, Dolly, and LLaMa may be a part of this layer. Microsoft is partnering with Hugging Face to bring a catalogue of curated open-source models to Azure.
While foundation models are powerful by themselves, they can be adapted for specific scenarios. For example, an LLM trained on a large corpus of generic textual content can be fine-tuned to understand the terminology used in an industry vertical such as healthcare, legal, or finance.
Microsoft’s Azure AI Studio hosts various foundation models, fine-tuned models, and even custom models trained by enterprises outside of Azure.
The foundation models rely heavily on the underlying GPU infrastructure to perform inference.
Orchestration – This layer acts as a conduit between the underlying foundation models and the user. Since generative AI is all about prompts, the orchestration layer analyzes the prompt entered by the user to understand the user’s or application’s real intent. It first applies a moderation filter to ensure that the prompt meets the safety guidelines and doesn’t force the model to respond with irrelevant or unsafe responses. The same layer is also responsible for filtering the model’s response that does not align with the expected outcome.
The next step in orchestration is to complement the prompt with meta-prompting through additional context that’s specific to the application. For example, the user may not have explicitly asked for packaging the response in a specific format, but the application’s user experience needs the format to render the output correctly. Think of this as injecting application-specific into the prompt to make it contextual to the application.
Once the prompt is constructed, additional factual data may be needed by the LLM to respond with an accurate answer. Without this, LLMs may tend to hallucinate by responding with inaccurate and imprecise information. The factual data typically lives outside the realm of LLMs in external sources such as the world wide web, external databases, or an object storage bucket.
Two techniques are popularly used to bring external context into the prompt to assist the LLM in responding accurately. The first is to use a combination of the word embeddings model and a vector database to retrieve information and selectively inject the context into the prompt. The second approach is to build a plugin that bridges the gap between the orchestration layer and the external source. ChatGPT uses the plugin model to retrieve data from external sources to augment the context.
Microsoft calls the above approaches Retrieval Augmented Generation (RAG). RAGs are expected to bring stability and grounding to LLM’s response by constructing a prompt with factual and contextual information.
Microsoft has adopted the same plugin architecture that ChatGPT uses to build rich context into the prompt.
Projects such as LangChain, Microsoft’s Semantic Kernel, and Guidance become the key components of the orchestration layer.
In summary, the orchestration layer adds the necessary guardrails to the final prompt that’s being sent to the LLMs.
The User Experience – The UX layer of the Copilot stack redefines the human-machine interface through a simplified conversational experience. Many complex user interface elements and nested menus will be replaced by a simple, unassuming widget sitting in the corner of the window. This becomes the most powerful frontend layer for accomplishing complex tasks irrespective of what the application does. From consumer websites to enterprise applications, the UX layer will transform forever.
Back in the mid-2000s, when Google started to become the default homepage of browsers, the search bar became ubiquitous. Users started to look for a search bar and use that as an entry point to the application. It forced Microsoft to introduce a search bar within the Start Menu and the Taskbar.
With the growing popularity of tools like ChatGPT and Bard, users are now looking for a chat window to start interacting with an application. This is bringing a fundamental shift in the user experience. Instead and clicking through a series of UI elements or typing commands in the terminal window, users want to interact through a ubiquitous chat window. It doesn’t come as a surprise that Microsoft is going to put a Copilot with a chat interface in Windows.
Microsoft Copilot stack and the plugins present a significant opportunity to developers and ISVs. It will result in a new ecosystem firmly grounded in the foundation models and large language models.
If LLMs and ChatGPT created the iPhone moment for AI, it is the plugins that become the new apps.